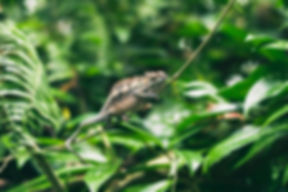
WHAT IS ABOUT
In recent years, the concerns on the risks of Artificial Intelligence (AI) are still growing. Safety is becoming increasingly relevant as humans are progressively ruled out from the decisions and control loops of intelligent systems. Indeed, the underlying AI techniques and algorithms, such as Generative AI (GenAI), Large Language Models (LLMs) and Machine Learning (ML), may compromise human values with harmful or untruthful responses. The technical foundations and assumptions on which traditional safety engineering principles are based, are inadequate for systems in which referred AI algorithms are interacting with the physical world and humans, at increasingly higher levels of autonomy. We must also consider the connection between the safety challenges posed by present-day AI systems, and more forward-looking research focused on more capable AI systems, up to and including Artificial General Intelligence (AGI).
This workshop seeks to explore new ideas on AI safety with particular focus on addressing the following questions:
-
How can we engineer trustable AI software architectures?
-
Do we need to specify and use bounded morality in system engineering to make AI-based systems more ethically aligned?
-
What is the status of existing approaches in ensuring AI and ML safety and what are the gaps?
-
What safety engineering considerations are required to develop safe human-machine interaction in automated decision-making systems?
-
What AI safety considerations and experiences are relevant from industry?
-
How can we characterise or evaluate AI systems according to their potential risks and vulnerabilities?
-
How can we develop solid technical visions and paradigm shift articles about AI Safety?
-
How do metrics of capability and generality affect the level of risk of a system and how trade-offs can be found with performance?
-
How do AI systems feature for example ethics, explainability, transparency, and accountability relate to, or contribute to, its safety?
-
How to evaluate AI safety?
-
How to safeguard GenAI/LLMs/ML?
TOPICS OF CONCERN
We invite theoretical, experimental and position papers covering any aspect of AI Safety including, but not limited to:
-
Safety in AI-based system architectures
-
Continuous V&V and predictability of AI safety properties
-
Runtime monitoring, guardrails, and (self-)adaptation of AI safety
-
Accountability, responsibility and liability of AI-based systems
-
Explainable AI and interpretable AI
-
Detection and mitigation of AI safety risks
-
Avoiding negative side effects in AI-based systems
-
Role and effectiveness of oversight: corrigibility and interruptibility
-
Loss of values and the catastrophic forgetting problem
-
Confidence, self-esteem and the distributional shift problem
-
Safety of AGI systems and the role of generality
-
Reward hacking and training corruption
-
Self-explanation, self-criticism and the transparency problem
-
Human-machine interaction safety
-
Regulating AI-based systems: safety standards and certification
-
Human-in/on/out of-the-loop and the scalable oversight problem
-
Mixed-initiative control frameworks
-
Evaluation platforms for AI safety
-
AI safety education and awareness
-
Experiences in AI-based safety-critical systems, including sectors like industrial, health, automotive, aerospace, robotics, among others
-
Approaches to comply with recent AI regulations and standards focusing on safety-related properties validation like robustness, stability, reliability and controllability